Machine Learning in Supply Chains
Get Some Quick ROI with Practical Use Cases
There is a lot going on with Machine Intelligence. With experimentation all around, use cases are popping up everywhere. As always, the value of a technology capability and the speed of adoption depends on picking a few promising opportunities and making a successful impact rather than trying out all possibilities. Credibility builds up quickly with real business impact and provides a tail wind for more experimentation. With my soft corner for improvements in operations using technology, I list a few use cases that could be quick wins. Possibly, some of you have tried these already (in which case would love to hear of your experiences) and some may be thinking of trying a few of them out (in which case would highly encourage it and await thoughts on why you would or wouldn’t think these as worthwhile opportunities).
At the current level of AI/ML maturity, the capability that has the potential to be the most effective is pattern recognition. Supply Chain is an area of the business where data is everywhere. It is in every transaction and if we watch carefully, that data is telling us things, on a daily basis, that humans are yet to notice, until it is loud enough to catch their attention. So, the ability to sense and respond is something we can put on steroids, if the human ability to understand patterns is combined with the computer’s ability to process mountains of transactional data to find those patterns. In embedding the logic, we don’t try to solve for causality. On the other hand, with correlations, we can let humans consider whether the correlation would imply a causality that needs a response. Let’s look at some simple use cases.
Forecasting:
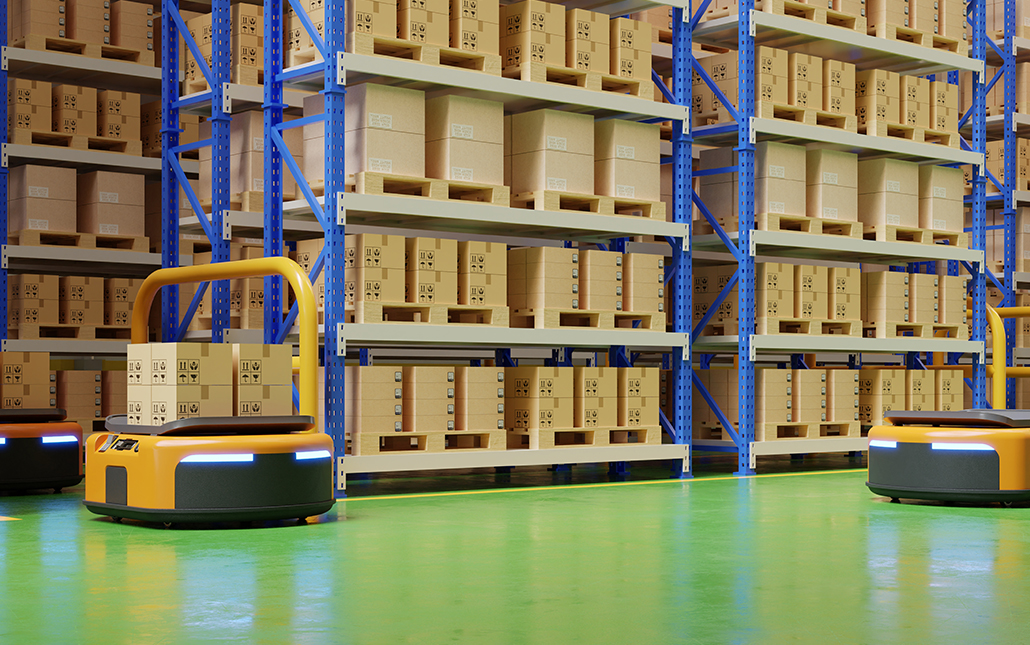
Every business needs a better ability to prepare for what lies ahead. Whether it is a hot product that we want to maximize sales opportunities for or optimizing the mix to improve capacity utilization or better margins, all businesses have a process (at varying degrees of sophistication) to enable this. It is the CFO and the finance organization that needs it most for this capability to be mature, for market guidance in public companies and for cash flow projections at smaller startups. For a long time, trend analysis was based on time series and regression tools that extrapolated past trends at varying levels of aggregation. But it was quantitative and dependent on user sophistication in picking the right leading indicators and parameters. Now with machine learning, we have a range of capabilities, with access to correlating quantitative trends with unstructured data. In the past when we projected trends for a SKU, we may not have factored in news updates of our competitor announcing a product launch in that category or our biggest customer changing their purchasing pattern to reduce quarter end inventory, until we heard it from the market through the salesforce. With a good ML model, we can start taking those early signs into our planning process and make the adjustments and fine tuning that can add another turn to inventory and improve capital efficiency.
Yield Improvement:
Managing and improving production yields is the lifeblood of a good manufacturing operation. Yields vary sometimes by operator, time in shift, machine settings, preventive maintenance, supplier quality variation, process quality variation etc. Identification of deviation and a quick response to fix the underlying issue is the driver of maintaining top yields and keeping a lid on manufacturing costs. Processing real time data acquired from various process tools and software, and analyzing them for variations is a key enabler in maintaining those high yield levels. You could pull a new operator for more training or schedule a preventive maintenance for a machine that is slowly trending to underperform ahead of seeing a decline in yields. Also automating visual inspection of parts to weed out defectives is ideally suited to the pattern recognition in imaging capabilities of ML enabled software. The earlier we are able to react the lower the waste and higher the productivity.
Shipping Costs:
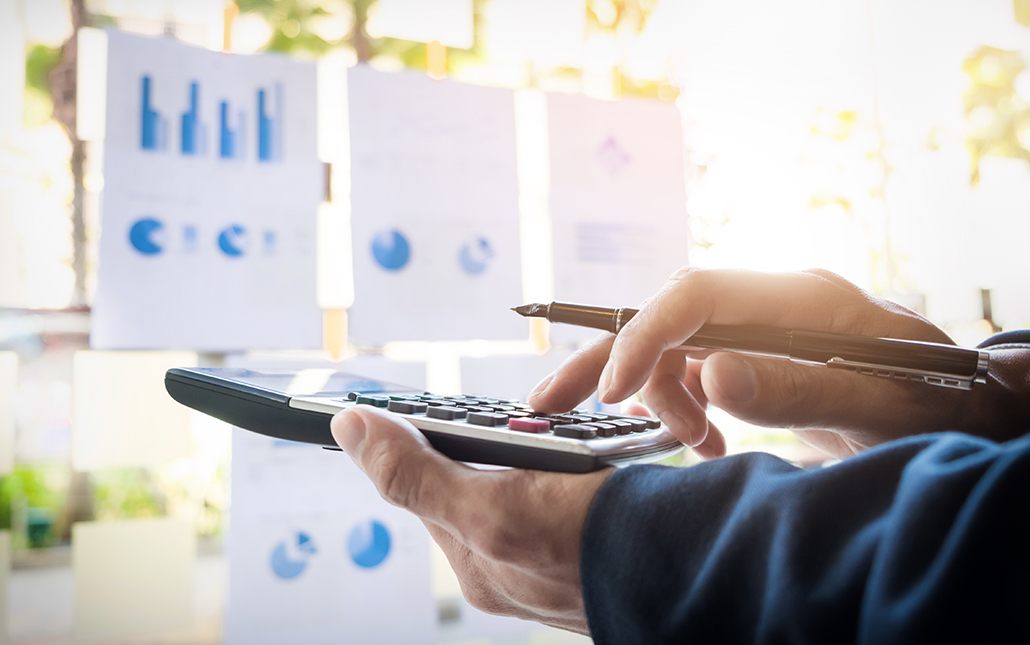
In a large volume multi-location logistics network, minor changes to things like order size optimization (i.e. combining orders instead of shipping separately to same destination), shipment mode changes vs. plan, opportunity to hold and maximize order quantity based on inbound updates, choosing shipment mode based on weather or other logistical events, etc. can be better done in a fast paced dynamic scenario with pattern recognition tools. Network optimization and inventory holding across the network can be adjusted more frequently with dynamic data instead of static analysis using historical data. Small tweaks can have a favorable impact on OM and SG&A over time if pursued diligently and carefully with the automated intelligence brought to the table with ML.
Sourcing Optimization:
Typical products have hundreds of components that are continuously changing in terms of prices, availability, new suppliers and sources, substitutable alternatives etc. Sourcing teams, charged with the responsibility of aiming for continuous cost improvement churn through websites, networks, trade journals, suppliers etc. to identify and drive those continuous improvement opportunities. In the past strategic sourcing programs were structured, periodic and took time to execute. They were frequently only number oriented. Here machine learning can analyze varieties of structured and unstructured data sources from trade journals to distributor catalogues, trade and tariff agreements to freight rates and landed cost impact, and do it on an ongoing basis to throw up potential suggestions to explore for the sourcing team. With a high-volume business even, a few opportunities can provide a ROI on machine learning quite quickly.
The above are some quick suggestions on exploring ML opportunities in the supply chain. I am sure there are many more active use cases, now that ML is becoming mainstream. As always, digital transformation involves applying emerging technologies to real world business problems to deliver business value. Hope to hear of other successful applications of Machine Learning in your contexts.
Share